On the 26th of May, Irene Teinemaa defended her PhD on “Predictive and prescriptive monitoring of business process outcomes“, in which she addresses the question on how to train, evaluate and use predictive models for outcome-oriented predictive process monitoring.
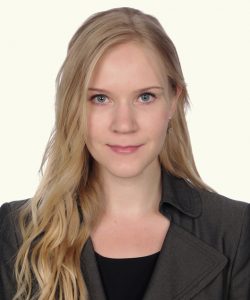
Every day, we are involved in various business processes. For instance, when booking a hotel, applying for a job, requesting a loan, or visiting a doctor. A business process is a collection of inter-related events, activities, and decision points that involve a number of actors and objects, which collectively lead to an outcome that is of value to a customer. A typical example is an order-to-cash process: a process that starts when a purchase order is received and ends when the product or service is delivered and the payment is confirmed.
Execution of a business process (called a case) yields a sequence of events. For instance, in an order-to-cash process, each purchase order gives rise to a case, which is represented by the sequence of events that are related to the same purchase order. Every event in the sequence contains various information, such as the type of the executed activity, the actors involved in that particular event, and the time of executing the event. Depending on the type of activity, the event can be associated with some additional information, such as the amount paid by the customer or the type or treatment assigned by the doctor.
Predictive business process monitoring techniques aim at making predictions about the future state of the cases. For example, a predictive monitoring technique may seek to predict the remaining execution time of each ongoing case of a process, the next activity that will be executed in each case, or the final outcome of a case, with respect to a possible set of business outcomes. For instance, in an order-to-cash process, the possible outcomes of a case may be that the purchase order is closed satisfactorily (i.e., the customer accepted the products and paid) or unsatisfactorily (i.e., the order was cancelled or withdrawn). Another set of possible outcomes is that the products were delivered on time (with respect to maximum acceptable delivery time), or delivered late.
Typically, predictive process monitoring techniques make use of machine learning in order to build models that are able to predict the various properties of an ongoing case. In outcome-oriented predictive process monitoring tasks, the goal is to make accurate predictions about the final outcome as early as possible, i.e. using only a few events from the beginning of the case. One of the main challenges that predictive process monitoring techniques need to tackle is how to encode the sequences of events in a way that is suitable for a machine-learning algorithm, without losing relevant information from the sequence. Another challenge comes from the heterogeneity of the cases. In particular, business processes are known to involve loops and parallel executions of activities, which yields a very large state space and highly variable sequence lengths.
The thesis “Predictive and prescriptive monitoring of business process outcomes” addresses the question of how to train, evaluate, and use predictive models for outcome-oriented predictive process monitoring. We start with a systematic review of existing methods, classify them into a taxonomy, and evaluate the techniques experimentally using a unified benchmark. Then, we extend the existing techniques by additionally making use of textual data associated with events. We evaluate the stability of predictions that are made for the same case over time. Finally, we propose an alarm-generation framework that notifies process workers if it is cost-effective to intervene in the process based on a certain prediction.
DSpace – https://dspace.ut.ee/handle/10062/63507