On the 26th of June, Elena Sügis defended her PhD on “Integration methods for heterogeneous biological data” in which she addresses the question of how to combine and analyze diverse biological data originating from various biological experiments and databases.
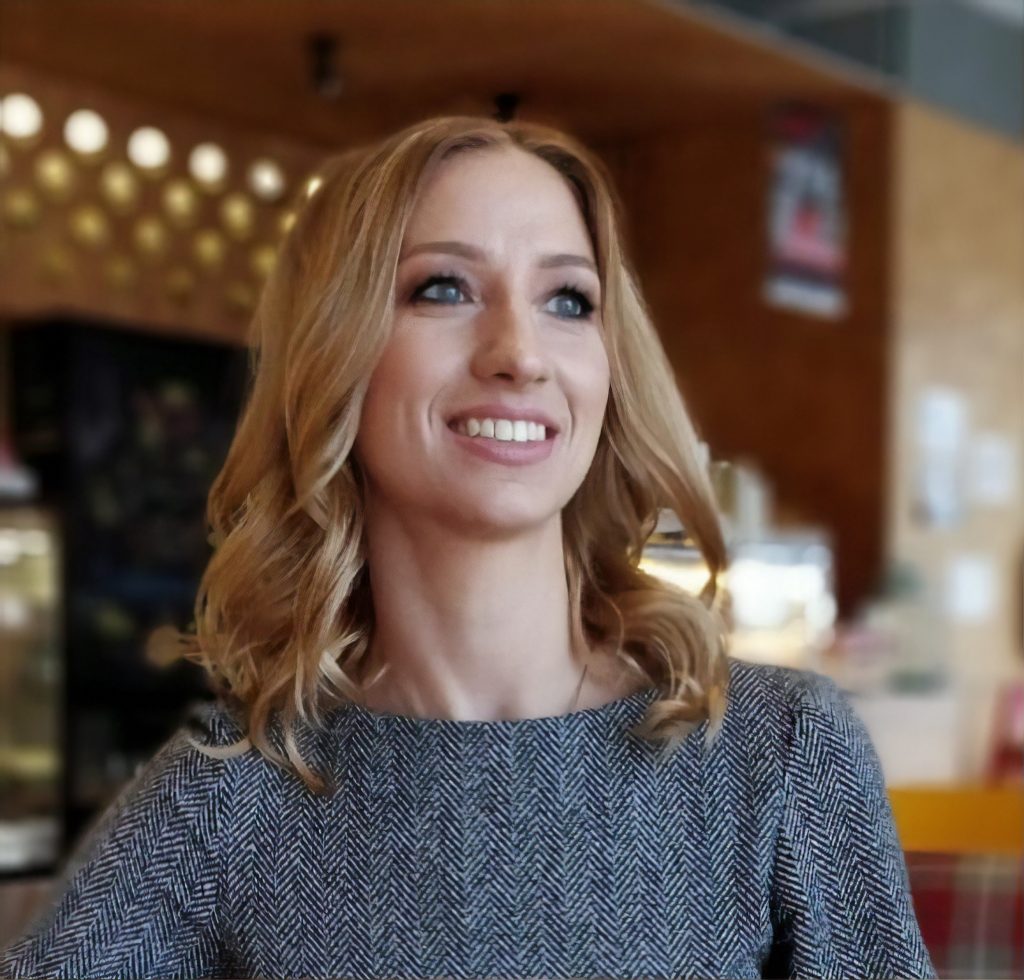
Importance of biological data integration
A fast advance in biotechnological innovation and decreasing production costs have led to the explosion of the experimental biological data, which is being produced every day in laboratories around the world. Individual experiments allow researchers to understand biological processes, like diseases from different angles. However, these discoveries provide only partial understanding when considered in isolation. In order to get a systematic view of the disease from these complementary data sets, it is necessary to combine them. The large amounts of diverse data requires an application of clever machine learning models that can help, for example to identify which genes are related to the disease or which drugs are toxic for early human development.
These models can be applied without making strong assumptions about the underlying biological mechanism. Artificial neural networks have gained popularity in different domains, for example allowing the development of self-driving cars, automatic speech translation, etc. They are also showing potential in application to large volumes of biological data. Additionally to the selection of the integration frameworks and analysis methods, there is a need for a composition of the reliable integrated data sets that researchers could effectively work with ensuring data quality for the subsequent analysis and reuse.
Key findings and impact
In this thesis, we demonstrate how to combine and analyse different types of biological data together in the application to three biological domains: Alzheimer’s disease, immunology, and toxicology.
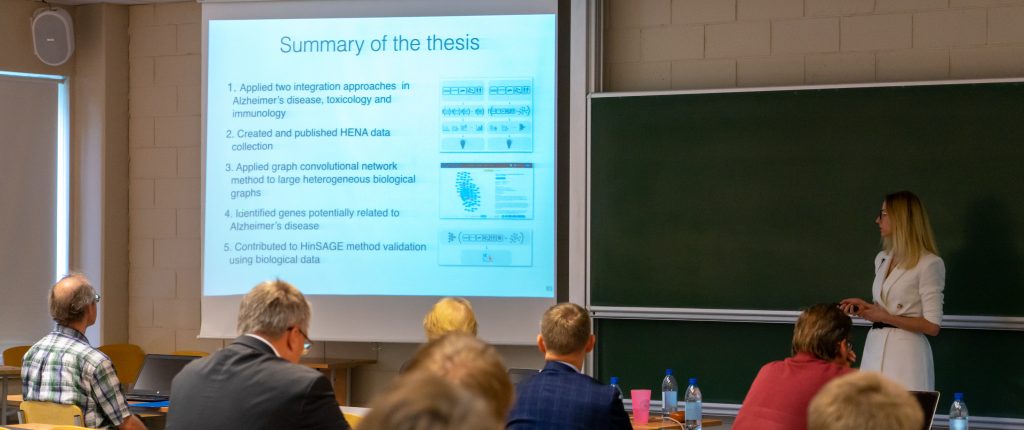
More specifically, we combine data sets related to Alzheimer’s disease into a novel heterogeneous network-based data set for Alzheimer’s disease (HENA). We then apply graph convolutional networks (GCN), a state-of-the-art deep learning methods, to node classification task in HENA to find the genes that are potentially associated with Alzheimer’s disease.
Combining patient’s data related to immune disease helps to uncover its pathological mechanisms and to find better treatments in the future. We analyse laboratory data from patients’ skin and blood samples by combining them with clinical information. Subsequently, we bring together the results of individual analyses using available domain knowledge to form a more systematic view on the disease pathogenesis.
Toxicity testing is the process of defining harmful effects of the substances for the living organisms. One of its applications is safety assessment of drugs or other chemicals for early development of a human organism. In this work, we identify groups of toxicants that have similar mechanism of action. Additionally, we develop a classification model that allows assessing toxic actions of unknown compounds.
International and local collaboration
This work is a good example of tight local and international collaboration between the Institute of Computer Science, University of Tartu (Estonia) and academic and industrial partners from various countries of the European Union. The toxicology studies were largely conducted together with the University of Konstanz (Germany). The immunology study was performed together with the scientists from Molecular Pathology Research Group, Institute of Biomedicine and Translational Medicine, University of Tartu and doctors from the Dermatology Clinic of the University of Tartu (Estonia). The large study of Alzheimer’s disease involved research groups from Swiss Institute of Bioinformatics (Switzerland), EMBL-EBI (UK), INSERM (France), TAU (Israel) and companies such as Hybrigenics (France), Quretec (Estonia) and many more.
DSpace – https://dspace.ut.ee/handle/10062/63816
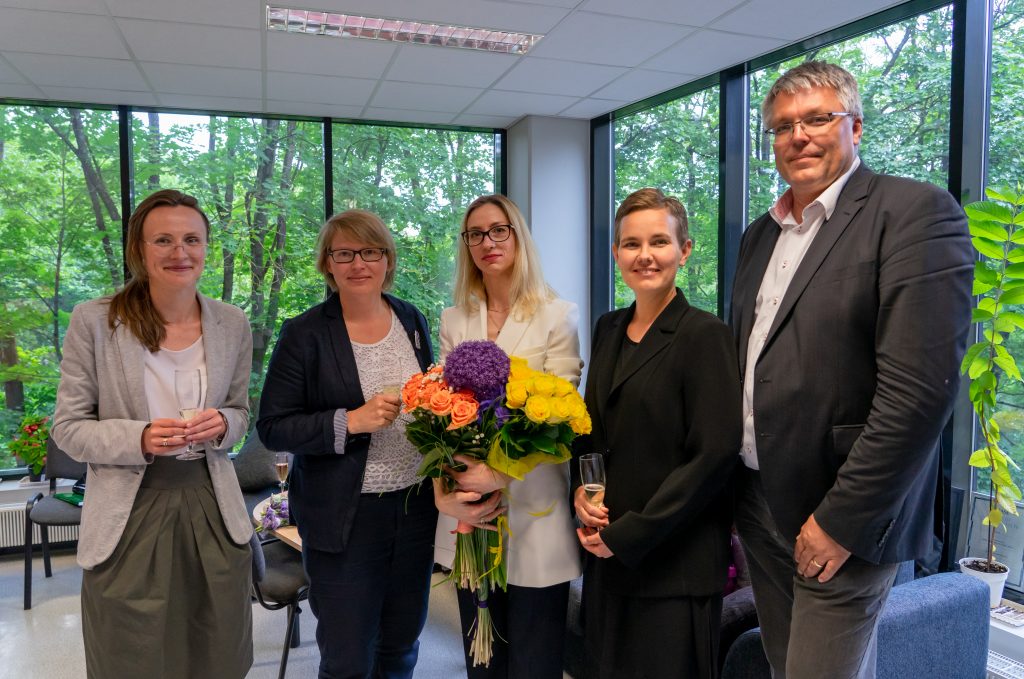