By Anastasiia Shevchenko
The competitive advantage of a manufacturing company consists of the relation between the delivery time, price, quality and flexibility. The customer’s expectation of the best relation between the above factors triggers the company’s need to constantly monitor its processes, making them more efficient. When identifying bottlenecks, it is important to maintain objectivity – not to make decisions based only on instincts, but to rely on data. This approach helps to prevent problems and errors that can be easily derived or confirmed by data analysis.
The Industrial Master’s Programme in IT, which brings together data science and manufacturing, uses the following approach when planning the launch of a collaboration:
- Definition – identifying and correctly formulating the problem that the manufacturing company wants to solve;
- Visualization – the parties think through and set expectations for what should be improved by solving the problem. Each party is an expert in its own field (the company in production and the partners/university in data science), which helps to maintain the emphasis on achieving an actual result, taking into account the time frame of the programme;
- Designing / planning the solution – the process of solving the problem is designed: who does what; what data and to what extent is needed; where this data comes from, etc.;
- Prototyping – building the initial solution;
- Testing – testing of the initial solution. Before the company invests on a large scale in the implementation of the solution, it is tested on a smaller scale whether the given solution meets the expectations formulated in the second stage.
It is important to emphasize that this is a process, not a one-off activity, meaning, if it turns out during the collaboration that the problem formulated in stage one does not correspond to reality, then it can and must be changed, regardless of the stage of the collaboration. This ensures the correctness, necessity and actuality of the created solution even after the end of the collaboration.
Stages one and two take place before the official start of the Industrial Master’s Programme in IT and the student joins in the third stage. At the same time, the onboarding period of the student must be taken into account, because for him the Industrial Master’s Programme starts with getting acquainted with the company (1-2 months) and only then moving on to the third stage together with the company.
PILOT PROJECT
Barrus is a wood industry company whose production cycle begins with the purchase of wood logs and ends with the production of finished products. The following video gives a good overview of the company and its production process:
Barrus, in collaboration with its long-term partner, Columbus Estonia, defined the problem that the manufacturing company is looking for a solution with the help of data science:
How to calculate the selling price of a new product based on the actual net cost?
Behind this problem is the desire to offer as correct a price as possible for the customer’s product, which, for example, will be produced in the future, and the solution is to create a predictive model based on machine learning. Together with the university researchers, it was determined that the problem can be solved using data science methods and solutions. As a result of the validation, a semester-based plan of the project was created, which makes it easier to monitor progress and make the necessary conclusions/changes.
The collaboration started in February 2021 and the activities for the first four months were as follows:
- In February, Richard Nõmm, the master’s student in the programme, was introduced to the work of the company as a whole. In the course of this, the student also identified other processes that, in his opinion, could find support in the implementation of machine learning. In order to keep the focus, these topics have been set aside at the moment and will be revisited if necessary and possible.
- In March, Richard was primarily involved in mapping data collection, i.e. identifying how data is collected and recorded today.
- In April, the focus was on the concept of net cost – how the net cost of a product forms and how to calculate it. Barrus and Columbus introduced Richard to different approaches, out of which the most suitable will be chosen in the coming months.
- In May, Richard spent a day in production to get acquainted with the production process in practice.
As of today, the first goals have been achieved and the project is progressing according to plan. The following aspects to consider are worth mentioning when planning such collaboration:
- In order to ensure that the project stays on schedule, the manufacturing company should have several people involved in the project. This helps the project to be less dependent on the availability of only one key person.
- The student must be proactive and independent. Thanks to Richard’s courage to communicate openly, great willingness to act and quick wit, the project has gone smoothly.
The next steps are:
- Validation of the concept of the net cost – this concept is the basis of the machine learning model (part of designing the solution phase);
- Finding a supervisor for Richard from the university – a person who would support with technical skills and knowledge in designing and creating a machine learning model (part of the prototyping phase);
- Building and testing a machine learning model (part of the prototyping and testing phase).
Finally, an overview of why Barrus decided to invest in solving the problem of calculating the selling price. The company’s production process can be presented in a simplified way as follows:
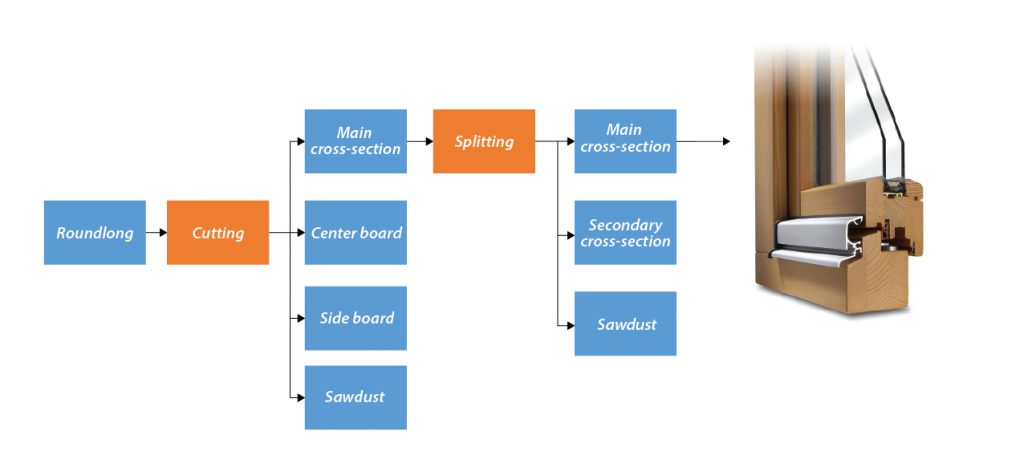
As it can be seen in figure, making a final product from a single log is a long process in which various by-products (sawdust, secondary cross-section, center board, knotted wood, etc.) are formed. All these by-products are valuable in the wood industry:
- Sawdust is sold or used for heat production;
- Secondary cross-section, center board and knotted wood can be used as the main cross-section in other products.
Taking all of these aspects into account makes it difficult to calculate the price of the final product, which, in our opinion, is not difficult for a well-designed machine learning model.
You can read the first part here.