While human average life expectancy has surged in the past century, a growing concern is a rising disease burden in the later stages of life. It is well known that lifestyle choices can help to prevent or delay disease onset, but once the disease has manifested, medical treatment (such as in the form of drugs) is usually sought after and applied. To provide cure however, there needs to be a sufficient understanding of disease processes. Particularly important is the identification of genes that are active in these processes – the disease-causing genes – as drugs could be developed to target the proteins encoded by such causal genes.
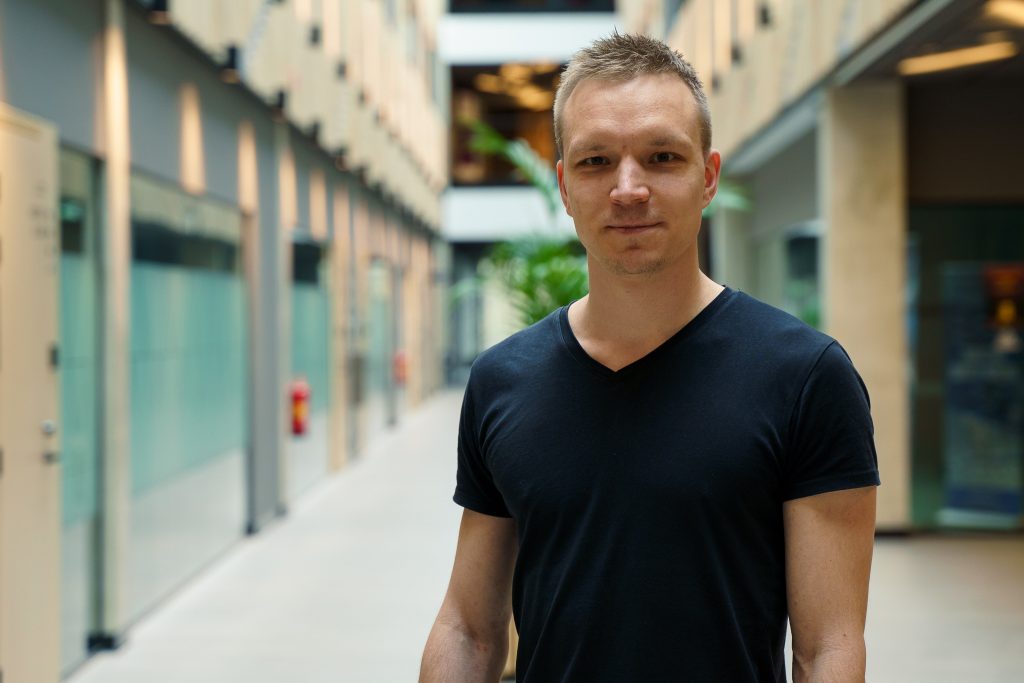
Discovering causal relationships between any two traits is often not straightforward. Lab experiments and randomized controlled trials (RCT) represent the main standard for doing so (several rounds of RCTs are necessary to verify the effectiveness of a new drug and bring it to the market) but can be time-consuming and expensive to undertake. Computational means and statistical analyses are obvious solutions to such inefficiency but come with their own considerations. Specifically, observational studies with mainstream association-based methods are very receptive to biases due to unjustified method assumptions and thus are not useful for finding disease-causing genes (we hypothesized that gene expression-complex trait associations are more likely to include either random noise or disease-induced changes in gene expression), even though they are often used for exactly this purpose. In genetics however, genotype information can be used as an anchor (instrument) for teasing out causality.
Genetic variants are fixed at birth and inherited following a random process. The genetic associations (genotype-phenotype relationships) are thus almost free of confounding factors, and are considerably less affected by biases in effect estimation and interpretation – after all, phenotype cannot cause the genotype. Exploiting this, if a gene expression trait and an outcome trait (e.g. disease) share an overlap in genetic bases, there is evidence for a causal interpretation between the two traits. Since genetic variants are utilized as instruments in making such inference, the corresponding method is termed Mendelian randomization (MR) after Mendel’s laws of inheritance. The randomness in genotype/instrument inheritance means that MR could even be considered as a natural computational extension to RCTs. In our research, we developed and adapted the methods of causal inference, in particular MR, to identify genes causally related to complex traits and diseases.
Since most human traits are polygenic, each genetic variant tends to have only a tiny effect on the outcome. This limits power and makes MR infeasible in smaller samples, particularly when there are many gene-outcome pairs to test for. We applied the principles of causal analysis to develop methodology for identifying causal genes in smaller samples (n ≈ 500), circumventing the low power of MR with structural equation models, applying MR only to previously prioritized set of potentially causal genes. We applied our methodology on the Estonian biobank data to ascertain the function of an inflammatory biomarker C-reactive protein, providing novel insight into its protective role in immune response – a result we validated in the lab.
In line with polygenicity of human traits, most genes tend to have an effect on many different phenotypes – a phenomenon called pleiotropy. Utilizing this domain knowledge, we extended the standard MR method into a multivariable approach by modeling causal effects of several related genes at the same time. In a hypothesis-free scan over the entire genome and 43 complex traits, we identified thousands of novel putative causal gene-trait relationships. We took an especially in-depth look into one specific disease-associated genomic region (16p11.2) and were able to pinpoint genes responsible for reproductive health – a result we once again validated in a lab environment.
The personalized medicine movement has gathered significant momentum in recent years. One of the major applications of precision medicine is to differentiate medical intervention strategies by sex – after all, most human traits exhibit sex specificity. Drugs are usually developed following a one-size-fits-all approach which has resulted in a higher rate of side effects in women compared to men. We investigated the sex-specificity of causal genes and whether statistical methods could be used to identify the differences. Using power analyses, we showed that sample sizes in the millions are required in the public domain to provide a definite answer to this question.
In conclusion, we showed the benefit of computational approaches in identifying disease-causing genes. While the final judgement needs to be delivered in experimental settings, computational methods facilitate fast and in bulk analysis which are likely to propel us to new medical discoveries.