On 23 December, Veronika Plotnikova defended her PhD thesis on “FIN-DM: A Data Mining Process for the Financial Services”, which investigated standard data mining frameworks, their usage across different industries and associated gaps. Based on the results, the new process model FIN-DM was developed to support practitioners to conduct data mining in the financial services.
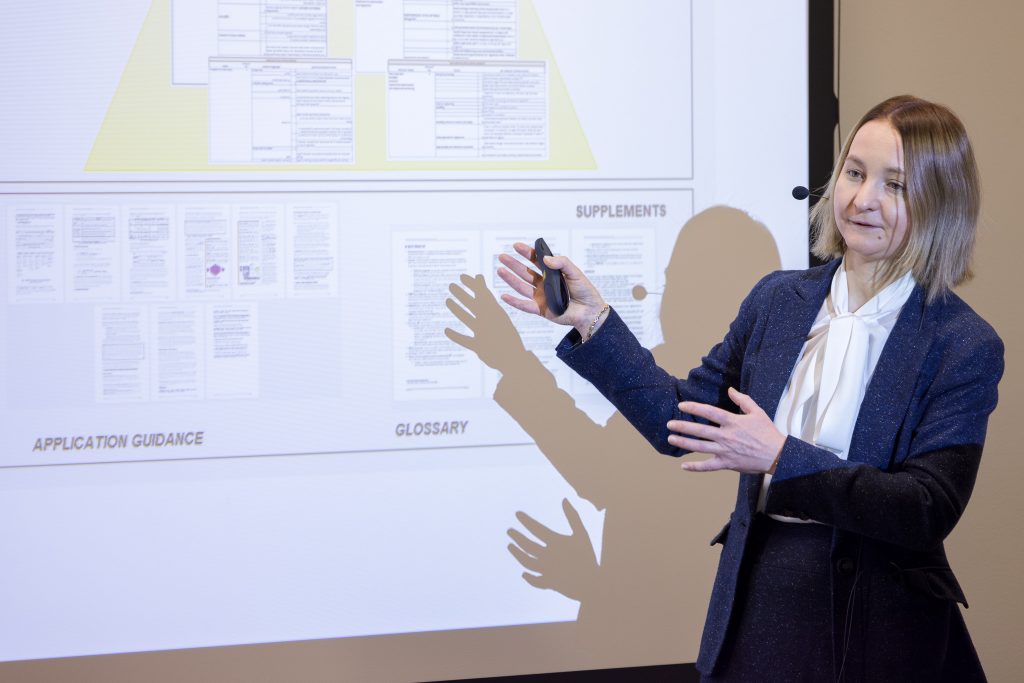
The adoption and use of data mining to support decision-making in organizations has grown exponentially over the last decade. In some industries, for instance, in the financial sectors, it has become an enterprise-wide practice. To ensure that data mining projects deliver the intended outcomes, organization use standard guidelines and process models to conduct data mining projects. One of the most well-known and widely adopted frameworks is CRISP-DM (Cross-Industry Standard Process for Data Mining). However, these standard processes and frameworks, and CRISP-DM in particular, are industry-agnostic, and therefore, do not meet industry-specific requirements. In some situations, such conditions are related to regulatory demands (e.g. privacy or risk management), and if overlooked, might result in company being non-compliant. In other situations, there might be inherent limitations of the data mining process, which reduce effectiveness of data mining projects and inhibit business value realization.
In this thesis, Veronika and her collaborators initially investigated how data mining frameworks are applied across various industries and financial services in particular. The research also involved a case study in the actual organization with extensive interviews with data mining practitioners. Analysis of the obtained data allowed to identify and catalog a number of gaps in the standard data mining process of CRISP-DM, including gaps specific to the financial services industry. Combining the design science approach with requirements engineering, the new data mining process, FIN-DM*, was designed and developed to address the given gaps. Importantly, the FIN-DM prototype was evaluated with the practitioners, and their feedback was documented and incorporated into the final model design. Veronika’s and collaborators’ proposed process model is valuable tool for practitioners as it addresses number of current challenges in data mining. In particular, how to conduct privacy-compliant data mining, tackle AI ethics issues and mitigate AI ethical risks in the data mining project. It also provides number of practical solutions to incorporate quality assurance and improve actionability of data mining outcomes.
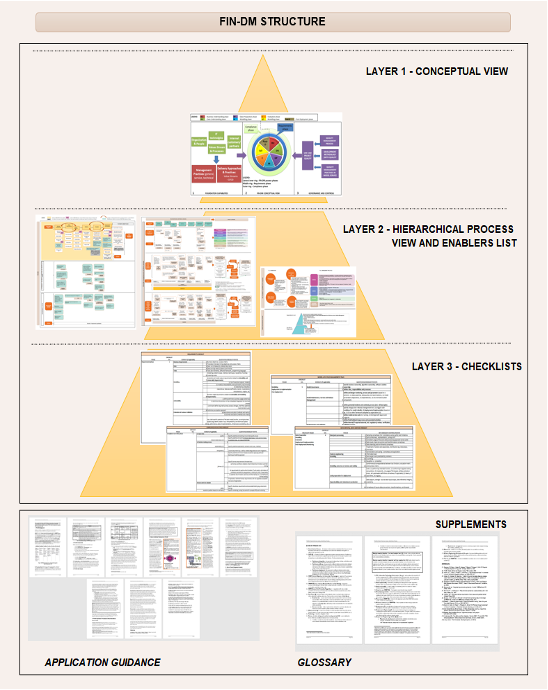
*- available on website www.fin-dm.info