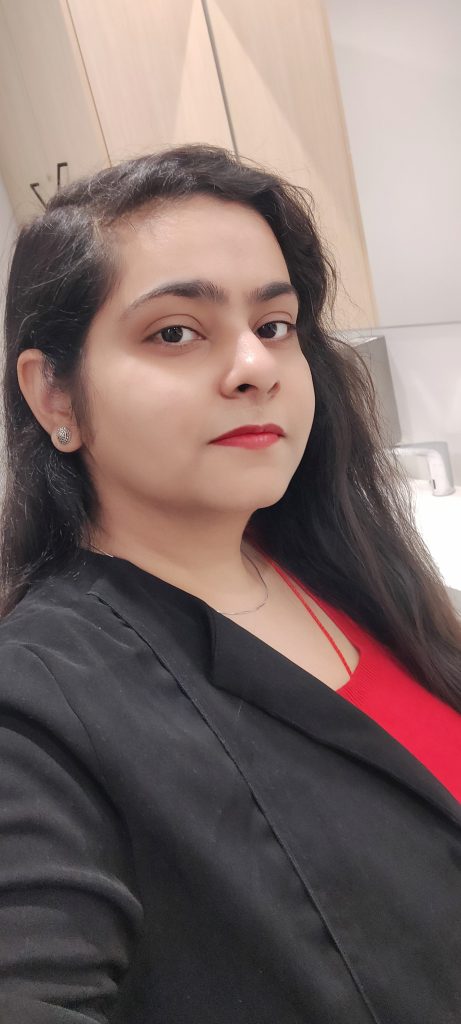
With the development of Online Social Media (OSM) platforms like Twitter and Facebook, the way individuals connect and exchange information has changed dramatically. These platforms have, however, also led to the problem of misinformation, which can quickly spread on a global scale, and once it has been disseminated, it can be hard to stop. It is to be noted that misinformation is an umbrella term that includes rumors, fake news, etc. This article examines the multifaceted approach for combating misinformation in the digital era, focusing on three key dimensions: characterizing misinformation content, developing a framework for identifying misinformation spreaders, and carrying out effective counter misinformation measures.
Characterization of Misinformation Content:
To understand the traits of misinformation posts and uncover the cognitive behavior and motivations behind spreading fake news, we proposed a characterization approach. This work is inspired by the idea that understanding the writing styles of such users can help understand cognitive behavior or identify the main motivations behind posting misinformation. By scrutinizing the linguistic characteristics of misinformation and non-misinformation posts, we can develop efficient automated methods for detecting and limiting the dissemination of fake news on social media platforms.
Through our linguistic analysis of the widely used publicly available PHEME-9 dataset of rumors and non-rumors tweets, we discovered a substantial difference between rumor and non-rumor psycho-linguistics source features, as well as between reply features. We discovered that rumor source tweets used more past tense words, prepositions and contained drives (motivation) related to reward, risk, and power. Whereas non-rumor source tweets had more present tense words, informal language, and had drives related to affiliation and achievement. We also explored the effectiveness of these features in predicting rumors. Specifically, we discovered that the ensemble-based Random Forest model, for all events, outperformed the other used models. As some machine learning models are black-box in nature, we utilized the SHAP AI Explainability tool to look for the features that are more important than the other features (Figure 1). This helped to comprehend which features are more important in classifying the tweets. For more details, please read our psycho-linguistic paper.
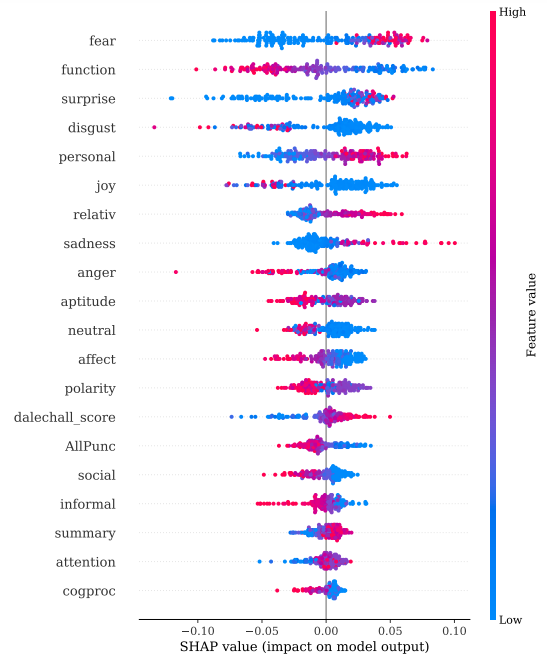
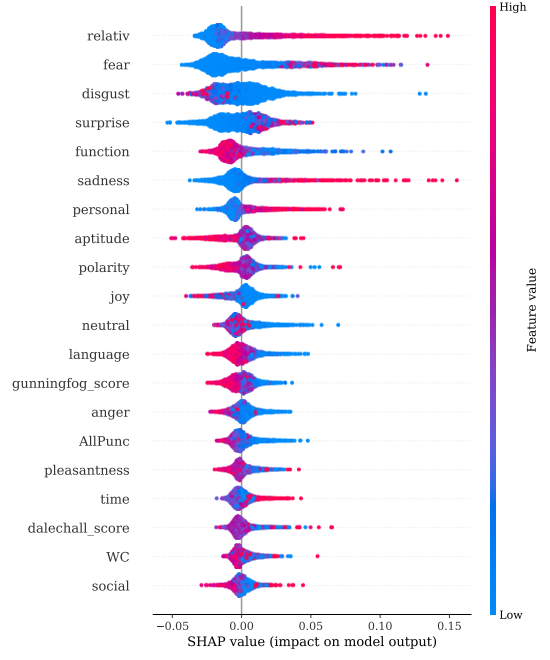
Figure 1: Charlie SHAP plot to determine feature importance.
Developing a Framework for Identifying Misinformation Spreaders:
Current techniques for finding misinformation posts on social media sites like Twitter frequently overlook user-level detection. It is insufficient to label a user as a disseminator of false information based solely on one post. Our research focused on the creation of an advanced automated classification system. This system seamlessly integrates data gathered from a multitude of posts and networks, ultimately yielding significantly more accurate results (Figure 2). We define rumor spreaders as those users who are often engaged in spreading rumors. Identifying possible rumor spreaders is important, as they could be the potential source of misinformation propagation. We use the PHEME dataset, which contains rumor and non-rumor tweets about five incidents that occurred between 2014 and 2015. In order to transform the tweets dataset into a rumor spreaders dataset, one of the techniques we utilize is the weak supervised learning technique, which is a branch of machine learning used to label the unannotated data using few or noisy labels in order to avoid the expensive task of manual annotation of the data. After the data transformation step, we leverage the following three distinct features for classifying possible rumor spreaders: user, text, and network features. In order to capture the network properties, we employ a Graph Neural Network (GNN) based approach for identifying possible rumor spreaders. By using this strategy, we were able to beat out the existing models currently in use and obtain a high F1-Score of up to 0.864. Refer to the paper for more details.
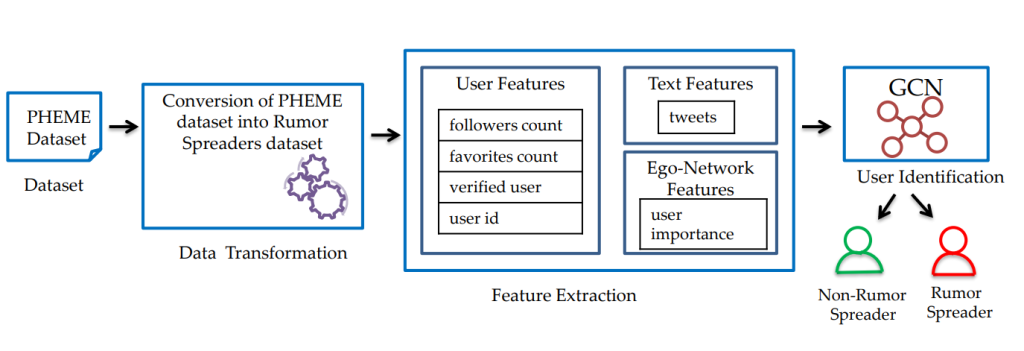
Figure 2: Framework to identify Misinformation Spreaders
Carrying Out Effective Counter Misinformation Measures:
We provide a plan of action strategy that involves an automated refutation of misinformation at scale in order to reduce the propagation of misinformation on social media. This strategy makes use of fact-checked data repositories and social media data. We investigate two complementary approaches to recognize misinformation posts at both early and later stages of propagation, with a focus on Twitter and the COVID-19 topic (Figure 3). Our suggested approach goes beyond the restrictions of existing approaches that can only handle one stage or the other. Our rebuttal system can prevent the spread of misinformation more effectively overall because it addresses both stages. Specifically, it leverages two publicly available datasets, viz. FaCov (fact-checked articles) and misleading (social media Twitter) data on COVID-19. The first identifies and reuses existing related but factual/non-misleading tweets by other users as recommended counter tweets. This is the baseline approach that can be used even when fact-checking websites are yet to carry out the vetting exercise for a given misinformation or while such articles are identified and matched by our second approach. The second recommends fact-checking articles (subject to availability) from various fact-checking sites. This provides users with verified, reliable, and more comprehensive information from reputable sources to counter the misinformation. By utilizing both of these automation complementary approaches in tandem, we create a scalable rebuttal pipeline to combat misinformation on social media.
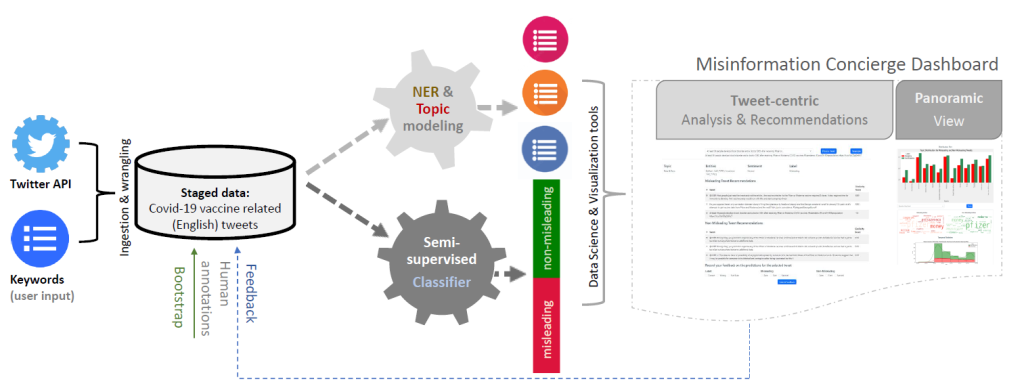
Figure 3: Automated Misinformation Rebuttal
Conclusion:
To summarize, the goal of my research is to create a thorough AI-based system to battle misinformation in the digital age. So far, my work involves focusing on three key dimensions, namely, characterizing misinformation content, developing a user-level detection framework, and implementing effective counter misinformation measures. We also give user privacy the utmost consideration in all of our work. Our study is important to the scientific community because it addresses the pressing issue of misinformation proliferation in the contemporary digital environment and provides a thorough strategy to combat it, ensuring a more educated and responsible use of information.