Protests and conflicts have become influential forces for bringing about change and shaping the political and social landscapes not only on a local demographic level but also on a global scale. In the modern Internet and online social media era, protests have increasingly utilized digital platforms to facilitate discussions, coordinate actions, and spread respective agendas. Despite this, there still needs to be a greater understanding regarding the interconnections between such political and the actions of individuals and organizations, particularly in Commonwealth of Independent States (CIS) countries.
Understanding a longitudinal change in language behaviour during Euromaidan Revolution in Ukraine in 2013
Despite the topic of protests being extensively studied, the question of language preference behaviour in online social media during the protests in multilingual countries is not covered enough. Most of the previous studies regarding language change either lack panel data or report simple aggregated statistics such as the number of comments in the particular language before and after some significant event.
To fill this gap, we analyze a dataset from the Facebook group called EuroMaydan that was explicitly created to facilitate the protest in Ukraine from November 2013 to February 2014. Moreover, our analysis follows the group even after the end of the protest till June 2014. This group had more than 300,000 subscribers when the data was collected. We use this panel data to test how users switched between two popular languages: the Ukrainian and the Russian languages. Please read the paper here.
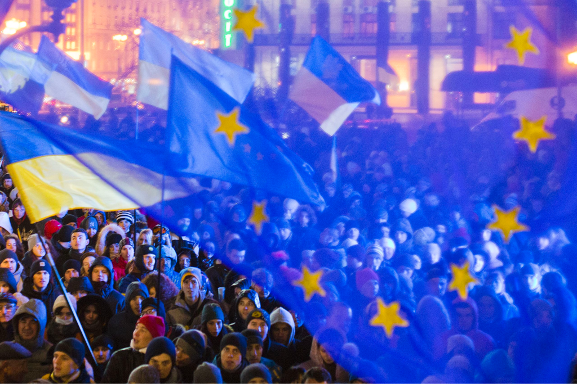
We show that active Ukrainian users changed their language preferences and used the Russian language more often after the end of Euromaidan. We also provide additional evidence that protesters use language rather strategically depending on the context instead of reacting to an ethnic or national mobilization. We also observed that the proportion of posts in Russian increased dramatically. Active users did not react to it by switching to identical language in their comments. Therefore, we suggest that administrators were not able to nudge the language behaviour of active users.
On the other hand, we observe the appearance of many new users who speak Russian in the data. It seems that active users reacted to new Russian speaking users by talking with them in Russian respectively. We also observe that the Ukrainian language is less sticky than the Russian language. In other words, even loyal Ukrainian speakers who were active before the end of the Euromaidan, are more likely to switch between languages after the end of the protest to react to new users (who often carried conversations in Russian). This finding is in line with the idea that Ukrainian activists use language strategically. They switch between languages depending on circumstances to facilitate communication instead of being nudged by national or ethnic mobilization. For more please read here.
Differentiable characteristics of Telegram mediums during protests in Belarus in 2020
Some platforms offer more than one kind of medium for communication, in particular, Telegram. We found that a lot of research literature focuses on either one or another medium or sometimes merges them together. In this research, we performed a comparative analysis of three communication mediums in this platform: channels, groups, and local chats during protests in Belarus in 2020.
We first collected a dataset which follows six months of protest, which allows us to study the temporal variation of online activism. We find that in Telegram, these three modes of communication are not equally important. Furthermore, they are responsible for different types of communication.
To investigate this question, we perform a three-stage analysis. Firstly, we analyze the most frequent words in each medium using WordClouds (Figures a, b, c). Secondly, we extract topics using Latent Dirichlet Allocation (LDA) and compare them among the mediums. We compare topics in two different scenarios. First, we analyze topics that we extract for the whole period of the data. Second, we also select the most important topics which overlap between any two mediums during their most active days (i.e. spikes). Finally, we analyze the context surrounding the names of politicians, protests and specific locations utilizing Word2Vec embeddings. We train three models for each medium and then for each noun. We find the top ten most important nouns using cosine distances among words’ embeddings. Then we infer the context surrounding using these ten words to find the main topics which users discussed.
We find out that users in groups mainly discussed announcements about national-level events. In contrast, local chats discussed local protests or demonstrations in particular neighbourhoods. What topics do users discuss in each medium? We observe that the topics vary by medium. Topics related to coordination were primarily raised in local chats (e.g., location and time of demonstrations), while channels and groups raised rather generic topics (e.g. news about the pandemic or Lukashenko’s behaviour). While these findings are not surprising, they show that the online communication during the Belarus protests was well structured. Therefore, one should be careful when studying online communication on Telegram and consider analyzing mediums independently instead of blending them in one dataset.
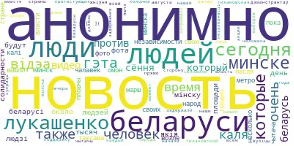
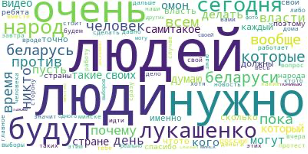
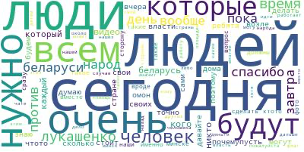
We also asked a question of whether users communicate distinctly in different mediums. In simple words, we wanted to understand if there are specific language patterns in each medium that can be easily recognized and predicted. It turns out that our models were able to predict messages only from channels. At the same time, we were not able to differentiate messages from local chats and groups. Our interpretation of this finding is that the administrators of channels used templates for communication, they referred to similar sources and copied similar news, and perhaps were engaged in some coordination. Thus, messages in channels were more homogeneous in their topics and style, and our models were able to recognize them as belonging to the same category. For more please refer here.
Identifying Events Targeted by Propaganda in Times of War: A Case of Russo-Ukrainian War
Disinformation and propaganda on social media can have severe consequences for individuals and society, particularly during conflicts and wars. Identifying events targeted by propaganda is crucial to mitigate the spread of disinformation and biased opinions. This can help people to be more sceptical of untrusted online news media and to question the honesty of the information they receive.
In this work, we propose an approach for detecting real-world events likely targeted by propaganda in social media. For this, we collected a large dataset of more than Telegram 300,000 posts from 10 channels from both sides of the conflict, belonging to both Ukrainian and Russian channels belonging to well-known politicians and popular mass media. These channels have a total audience of 10 million subscribers. In addition to our collected posts from Telegram, we obtained comprehensive and reliable datasets of external events by referring to the timeline of the 2022 Russian invasion of Ukraine, available in the Russian and Ukrainian languages in Wikipedia.
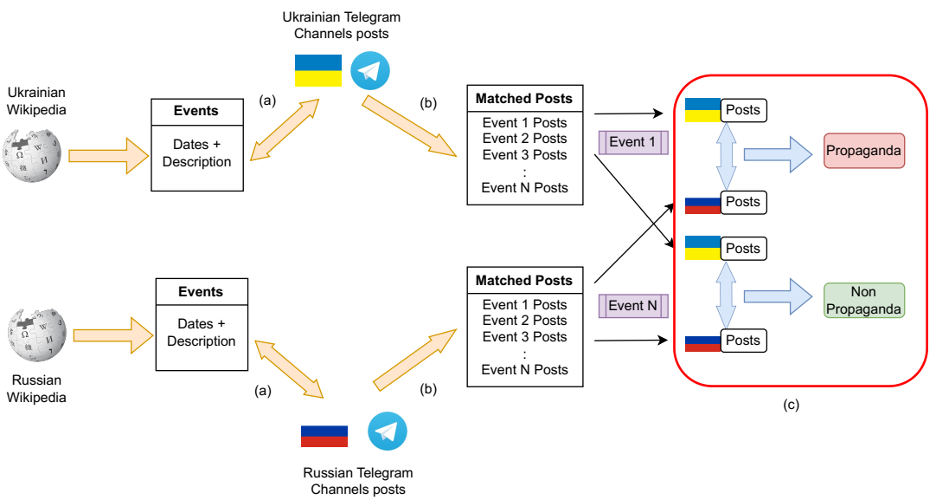
The first step is identifying posts from Ukraine and Russia related to a specific event. Next, embeddings are extracted for each post using a pre-trained language model. We used a multilingual Sentence-BERT approach that was trained in more than 50+ languages, including Ukrainian and Russian. The cosine similarity is then calculated between each possible pair of posts, one from Ukraine to the other from Russia. We take the average of the cosine distance and rank the events in descending order by cosine similarity.
We observe that most of the posts, with high dissimilarity, relate to major battles such as the Battle of Bakhmut or the Battle of Soledar, which have not only strategic but also political advantages for both sides. The analysis shows that events describing particular war clashes, specifically those containing the word “fight,” have a higher similarity to the rest of the events. Conversely, events such as the Bucha massacre, the rocket attack on a shopping centre in Kremenchuk, and the sinking of the ship Moscva were found to have the lowest similarity and alignment among posts on channels from the two countries.