On the 26th of August, Tõnis Tasa defended his PhD on “Bioinformatics approaches in personalized pharmacotherapy”. Tõnis’s thesis focuses on addressing problems in multiple sub-fields of personalised medicine and aims to illustrate that data for precision medicine emerges from different sources.
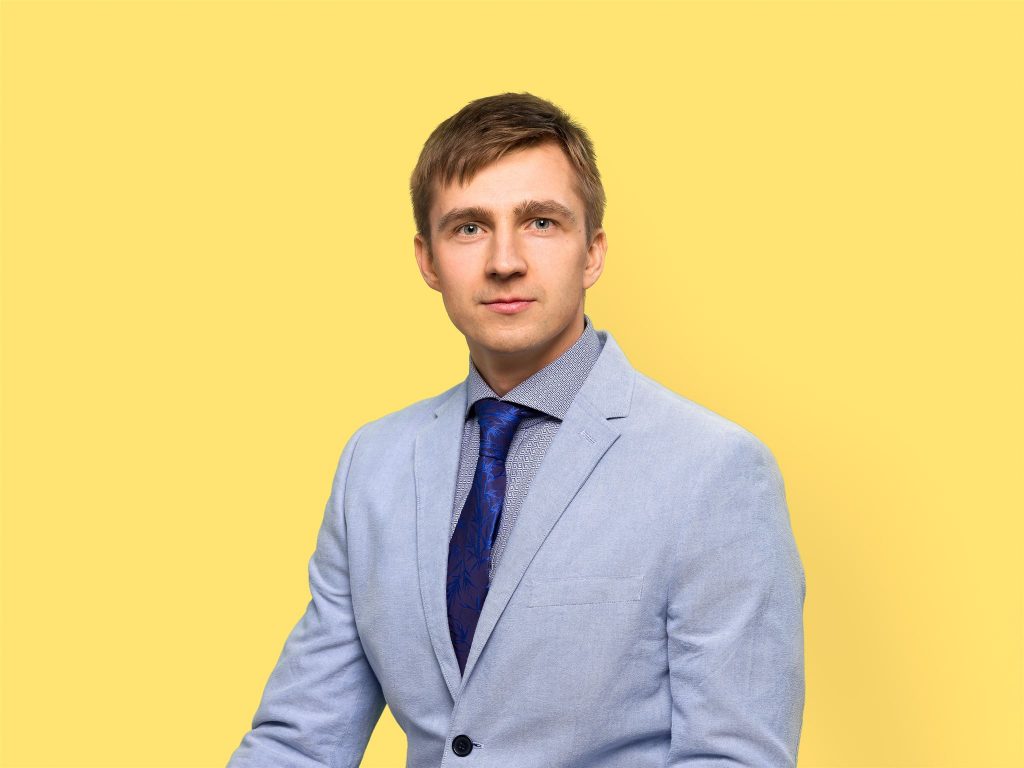
Conventional therapeutic medicine tackles pathologies with chemical, surgical or radiation-based interventions. Clinicians currently use basic patient characteristics such as age and sex in intervention decisions but not much regard is still given to other individual patient attributes due to lack of knowledge about patient biology and availability of such methods that would be guided by patient information.
Precision medicine is a broad concept that covers a variety of approaches that help doctors tailor the treatment to a specific patient. Essentially, more characteristics besides the most basic traits are used to inform medical decision-making. These new types of biological data had previously been unavailable, but rapid advances in genomic sequencing technologies have massively increased the amount of available biological information.
In fact, precision medicine is often primarily associated with genetic information explaining health outcomes. Certainly, DNA is a rich source of information for treatment individualisations that can guide the assignment of drug prescriptions. Metabolism of many drugs contains a handful of key genes where alterations cause effects that are large enough warrant changes in drug administration. Accounting for genetics can pre-emptively avert unwanted outcomes such as toxicity or adverse drug effects.
Genomic biobanks contain biological samples that provide the foundation for all population-based studies, and Estonia has a fairly developed electronic health infrastructure. Major work done by institutions such as Estonian Genome Center, Estonian e-Health Foundation, Health and Welfare Information Systems Centre and many of the hospitals, on collecting and managing biological data, provides a great source to study genomic and health data on a population scale.
A still on-going major task in genetics is to pinpoint genetic variation that manifests drug effects. One of the main workhorses for studying preliminary correlative relationships between genetics and any measurable traits is the genome-wide association analysis (GWAS) that is a pretty robust and scalable approach for any trait-DNA relationships.
A follow-up of a GWAS analysis often consists of an extended number of steps that aim to bring some biological context to preliminary findings. Some of these steps are becoming serious bottlenecks as the scale of genotypes and phenotypes continues to increase. One such type of analysis is the visual assessment of significance estimates from GWAS in context of other variants in nearby genomic regions. It is usually performed by manual inspection of plots for their subjective interest.
Usually, for most human traits genetic data is not the complete picture. Drug metabolism is difficult to predict because all sorts of biological differences cause variability in patient responses and for many traits, common variants do not explain enough variability to be clinically useful. Therefore, other types of biological data provide an extra dimension.
In therapeutic drug monitoring, drug concentrations from patients are used to adjust treatment so to make it more successful. This relies on drug concentrations being a good proxy towards estimating the drug effect. The key here is to link concentrations with some pharmacological indices and their respective values that in turn correlate with treatment outcomes. If an accurate model of concentrations can be related to an index that associates with clinical outcome of interest such as efficacy or toxicity then interventions from changed dosing provide a straightforward approach to modification of treatment.
This thesis aims to illustrate that precision medicine emerges from different sources.
To address the growing need for an actionable therapeutic monitoring tool that enables execution of pharmacokinetic knowledge, we have developed a precision dosing tool, DosOpt. This tool allows optimisation for individualised doses based on previously measured in-treatment drug concentrations for a drug called vancomycin in pre-maturely born children. It was externally evaluated in a retrospective dataset of vancomycin patients from Tartu University Hospital from 2010-2015.
Secondly, we used population-based genetic information from the Estonian Genome Center with linked drug prescription and diagnosed illness data from population registries to test and validate known associations between genetics and drug-related adverse effects. We characterised pharmacologically important genetic variation in Estonian population and found a completely novel association in CTNNA3 gene that increases the prevalence of adverse drug effects in patients with prescriptions for oxicams.
The previous study led to acknowledging the need for more automated detection of “interesting” visual peaks. Therefore, we set out to streamline candidate genomic area selection with automated methods for downstream processing. Our tools, Cropper and Manhattan Harvester, automate genomic peak detection based on GWAS summary statistics and assign quality scores by emulating the subjective assessment of human evaluators. This considerably decreases the time analysts spend on assessing plots.