On June 21, Liis Kolberg defended her PhD thesis on “Developing and applying bioinformatics tools for gene expression data interpretation” which provides convenient tools that simplify the work of biologists and saves their time.
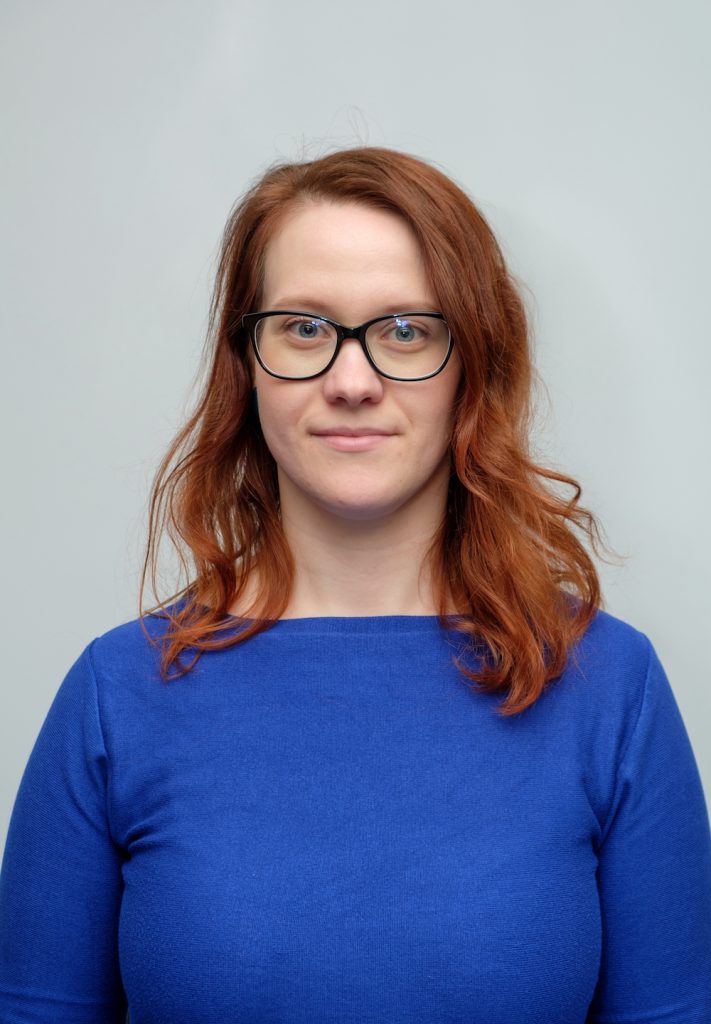
Modern technologies enable researchers to simultaneously measure the expression levels of all genes under different conditions and in different groups of people. For example, gene expression is measured in cancer and normal human tissues. The result of such an experiment is typically a high-dimensional gene expression matrix that can include expression levels of tens of thousands of genes across hundreds of samples. For example, such studies aim to find genes whose expression is aberrant in cancer and thus may contribute to its development. For this purpose, groups of genes with similar expression profiles are searched from these data using different data mining methods and statistical tests. Next, to better understand these groups, already known information about the genes is harnessed to elucidate their common functions and identify the biological processes where these genes’ products are involved. Thus, new functions of less-studied genes or new genes related to the studied disease can be found. However, such analyses require applying several methods and performing numerous statistical tests. For this reason, bioinformaticians develop tools that enable researchers to perform these tasks more efficiently.
The aim of Liis’s thesis is two-fold. Firstly, to develop efficient and easy-to-use bioinformatics tools for gene expression data interpretation that enable biologists to perform a standard analysis without requiring extensive programming from their side. Thus, these tools save the time of the researchers. The second aim of the thesis is to apply these tools to interpret genetic variants that affect gene expression levels.
First, Liis and colleagues extended g:Profiler, a web tool that finds significant intersections from the descriptions of gene lists. These intersections are found using the existing knowledge of the functionality of the genes and performing statistical tests to evaluate the enrichment of known functions in the given gene list. They also developed an accompanying R package to enable researchers and other bioinformaticians to include g:Profiler functionality into their analysis pipelines. This package has received great interest from the scientific community. On average, 155 000 queries per month are performed using this package. Furthermore, it has been included in external packages and is taught in several workshops. In addition, a web tool called funcExplorer (https://biit.cs.ut.ee/funcexplorer) was developed that groups genes with a similar expression profile, taking into account the descriptions found with g:Profiler. Thus, funcExplorer detects biologically meaningful gene groups that the user can directly interpret. Among other functionalities, the emphasis was put on presenting and sharing the results of the tools developed in this thesis. Interactive plots generated by these tools allow users to obtain a visually appealing overview of the data and the analysis results.
In her work, Liis also approached the problem from a different angle and applied the developed tools to carry out her own scientific study. First, funcExplorer was used to detect gene groups with a similar expression. Next, genetic variants that influence the expression of these genes were identified. Finally, g:Profiler was used to interpret these gene groups and thus the genetic variants that affect them. As a result, besides confirming previously known associations, this large-scale study described a novel association. Therefore further illustrating the usefulness of g:Profiler and funcExplorer on solving scientific problems. Furthermore, using her own tools in a practical analysis provided a fresh perspective on these tools and revealed some missing but useful features.