On March 23, Adriano Augusto defended his PhD thesis „Accurate and efficient discovery of process models from event logs„ in which he provided an overview of the available algorithms to perform automated process discovery. They identified deficiencies in existing algorithms, and proposed a new algorithm, called Split Miner, which is faster and consistently discovers more accurate process models than existing algorithms. It was the first PhD in the University of Tartu, which was defended online!
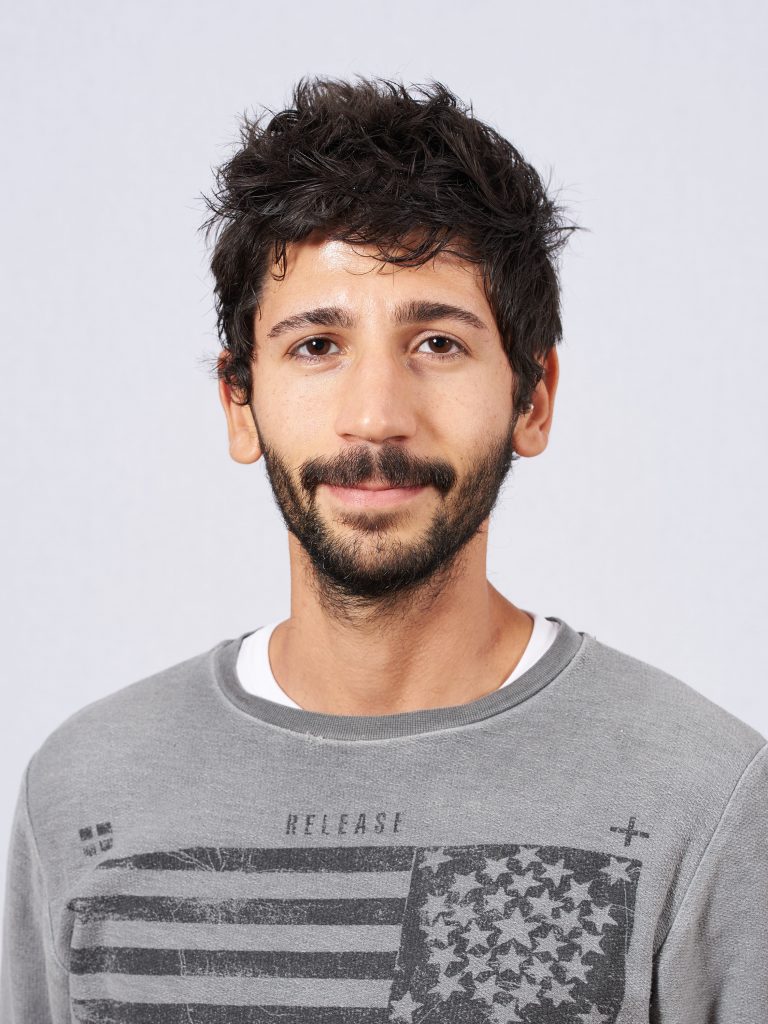
Every day, companies’ employees perform activities to provide services (or products) to their customers. A sequence of such activities is known as a business process. In other words, a business process is what a company does to provide a service or a product to a customer. The quality and the efficiency of a business process directly influence the customer experience. In a competitive business environment, achieving a great customer experience is fundamental to be a successful company. For this reason, companies are interested in identifying their business processes to analyse and improve them.
To analyse and improve a business process, it is necessary to document it. Business process documentation can have different forms, for example, textual (e.g. natural language process description) or graphical description. This thesis focuses on graphical representations of business processes, known as business process models. Drawing such process models manually can be time-consuming because of the time it takes to collect detailed information about the execution of the process. Indeed, traditional process discovery and modelling methods include: interviews of the process participants (i.e. the employees executing the business process), or direct observation of the process execution. On top of that, manually drawn process models are often incomplete because it is difficult to uncover every possible execution path in the process via manual information collection.
In recent years, following the design and development of advanced information systems and software technologies, a new method for discovering and modelling business processes came into play: Automated Process Discovery. Automated process discovery applications allow business analysts to exploit process’ execution data recorded by enterprise information systems to automatically discover process models. Discovering high-quality process models is extremely important to reduce the time spent enhancing them and to avoid mistakes during process analysis. The quality of an automatically discovered process model usually depends on both the input data and the automated process discovery application that is used.
In this thesis, first, we explore the currently available automated process discovery applications, to identify what are their strengths and limitations. Precisely, we provide an overview of the previous research studies that proposed applications to perform automated process discovery, we compare these applications by running our designed benchmark, and we provide an in-depth analysis of the benchmark results that highlight the strengths and limitations of the existing automated process discovery applications.
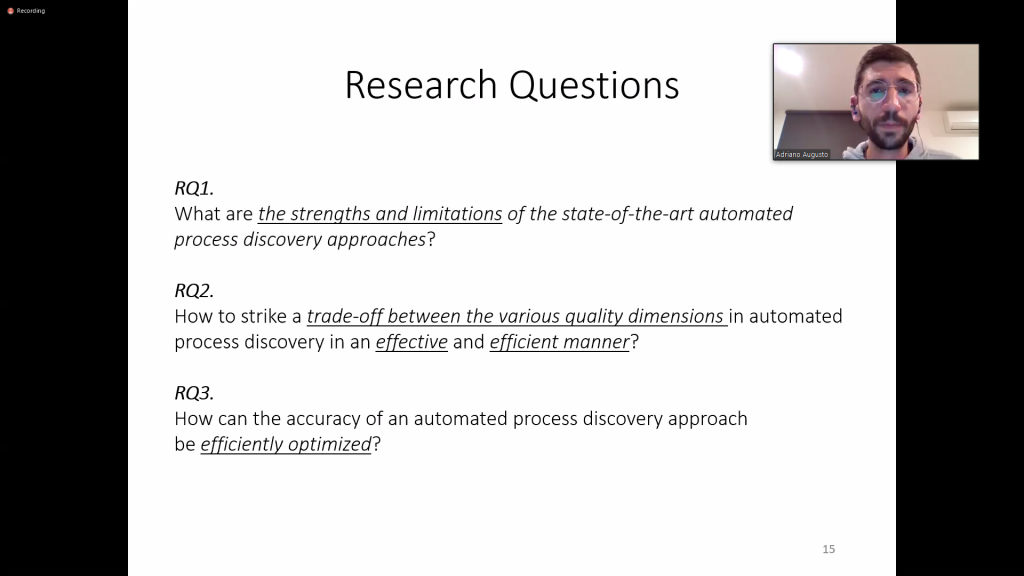
Following the results of our benchmark, the thesis describes new algorithms that can overcome the limitations identified in existing automated process discovery applications. We combine our new algorithms into an automated process discovery application called Split Miner, and we show that it is faster than existing applications and consistently more accurate.
Furthermore, we propose a new method to measure the accuracy of automatically discovered process models in a fine-grained manner, yet approximate. Such an approximation is required to improve the speed during the analysis of the accuracy of automatically discovered process models. We show that our accuracy measures provide results that are in-line with those outputted by existing accuracy measures while outperforming the latter in terms of time required to calculate the accuracy.
Finally, we leverage our new accuracy measures to design an optimization method that can improve the quality of a specific subset of automated process discovery applications, which also include our Split Miner. We show that by applying our optimization method, the automated process discovery applications can discover process models having an accuracy higher than those discovered without our optimization method, at the cost of a small increment of the execution time.